Speaking Volumes: AI Breaking Barriers for the Deaf and Hard of Hearing
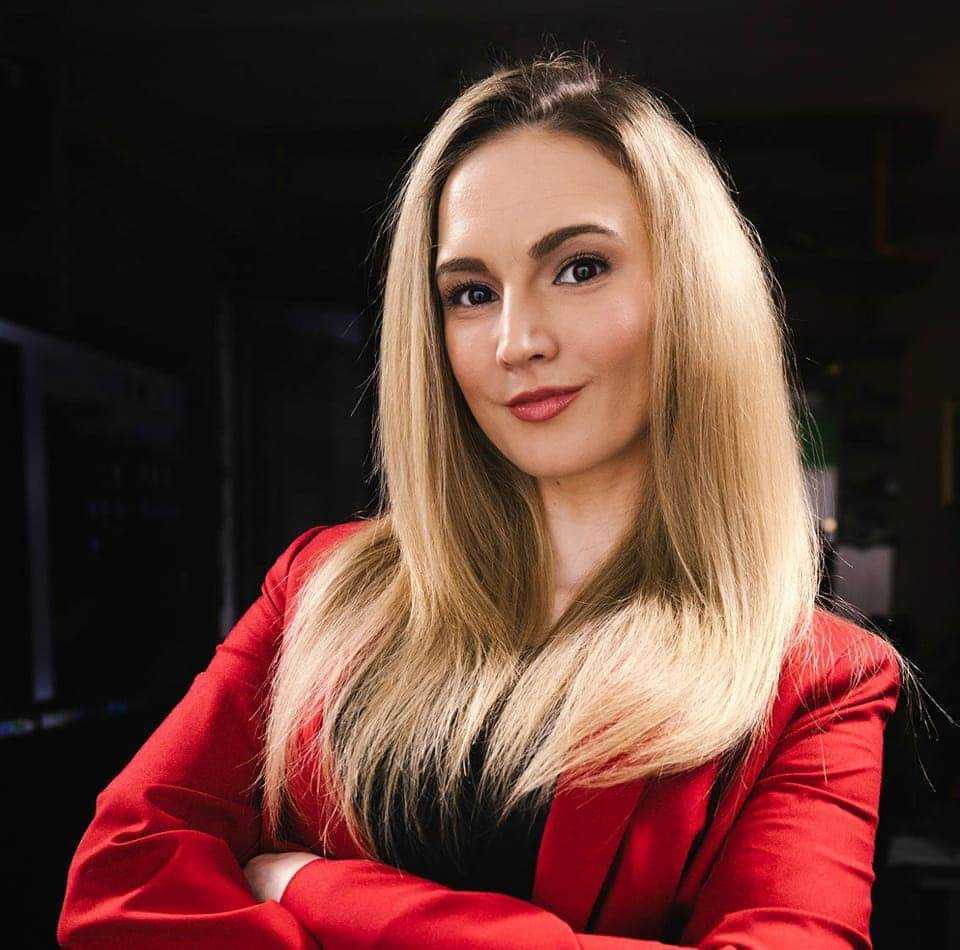
Patricia Butina
Marketing Associate
Published:
November 15, 2024
Topic:
Insights

Artificial Intelligence (AI) fundamentally transforms how we approach accessibility, especially for the deaf and hard of hearing community. With AI’s advanced algorithms and intelligent use of machine learning, it’s helping to break down long-standing barriers in communication and daily life. Understanding how these technologies work can give us a deeper appreciation of their impact and the new opportunities they offer.
Here’s an in-depth look at some of the AI-driven technologies that are pushing accessibility forward.

1. Automatic Speech Recognition (ASR): Translating Spoken Language to Text
Automatic Speech Recognition (ASR) is a critical tool for making spoken language more accessible to the deaf community. ASR systems are designed to convert speech into text in real time. But how do these systems work?
The Mechanics of ASR
- Capturing and Digitizing Sound: It starts with a microphone capturing audio, which is then broken down into digital sound waves. The system divides these sound waves into small segments, or frames, to capture even the tiniest phonetic differences.
- Feature Extraction and Analysis: From there, the AI identifies and extracts critical characteristics like frequency and pitch. These features are essential because they help the system differentiate between different sounds. One common technique used here is Mel-Frequency Cepstral Coefficients (MFCCs), which reflect how human ears process sound.
- Mapping Features to Phonemes with Acoustic Models: The AI then compares these features to pre-trained models that match them with phonemes—the minor units of sound that makeup words. Deep learning models like Hidden Markov Models (HMMs) or Deep Neural Networks (DNNs) are trained to recognize these phonemes across different accents or speaking speeds.
- Understanding Context with Language Modeling: To ensure the phonemes it recognizes make sense, the system uses Natural Language Processing (NLP) to apply grammar rules and understand the context. This step helps the AI differentiate between words that sound alike or fit grammatically in different situations.
- Real-Time Display of Transcribed Text: Finally, the system assembles these phonemes into readable text that instantly appears on a screen. This quick process allows ASR to provide live captions for videos, calls, and more.
Practical Uses of ASR
Educational institutions like the Rochester Institute of Technology (RIT) already use ASR tools to provide live captions during lectures. Meanwhile, apps like LiveTranscribe and InnoCaption use ASR to enable real-time captioning for conversations and phone calls, making everyday interactions more accessible for the deaf and hard of hearing.

2. AI-Powered Lip-Reading: Converting Visual Cues to Speech
For those who rely on lip-reading, AI is opening up new possibilities by combining computer vision with deep learning to recognize spoken language visually. While traditional lip-reading is tricky to master, AI is proving to be a game-changer.
How Lip-Reading AI Works
- Capturing Video and Extracting Visual Features: Lip-reading AI begins by capturing a video of the person speaking. It then focuses on the mouth and tracks changes in lip shape and movement. The AI then uses Convolutional Neural Networks (CNNs) to extract visual features from each video frame.
- Analyzing Temporal Changes: Each frame is treated separately, but to understand speech, the AI must examine the entire sequence of frames. This is where models like Recurrent Neural Networks (RNNs) or Long Short-Term Memory Networks (LSTMs) come in, helping the AI recognize patterns in lip movements over time.
- Linking Visual Features to Phonemes: Using a pre-trained model, the AI maps the visual features it extracts to specific phonemes. This process is similar to ASR but relies purely on visual data.
- Adding Context with NLP: Lip-reading AI also uses Natural Language Processing (NLP) to interpret context and improve the accuracy of its predictions. This means the AI can make educated guesses about words based on the visual cues it’s seeing and the context of the conversation.
- Filling in the Gaps with Predictive Learning: When lip movements are unclear or partially hidden, the AI uses predictive algorithms to infer the most likely words based on their training and context.
Applications of Lip-Reading AI
Google has made notable strides with its AI-powered lip-reading system, which achieves an accuracy rate of over 46%, considerably higher than most human lip-readers. This technology could eventually be integrated into hearing aids, giving users an additional tool for understanding spoken language in noisy environments or situations where audio is unclear.
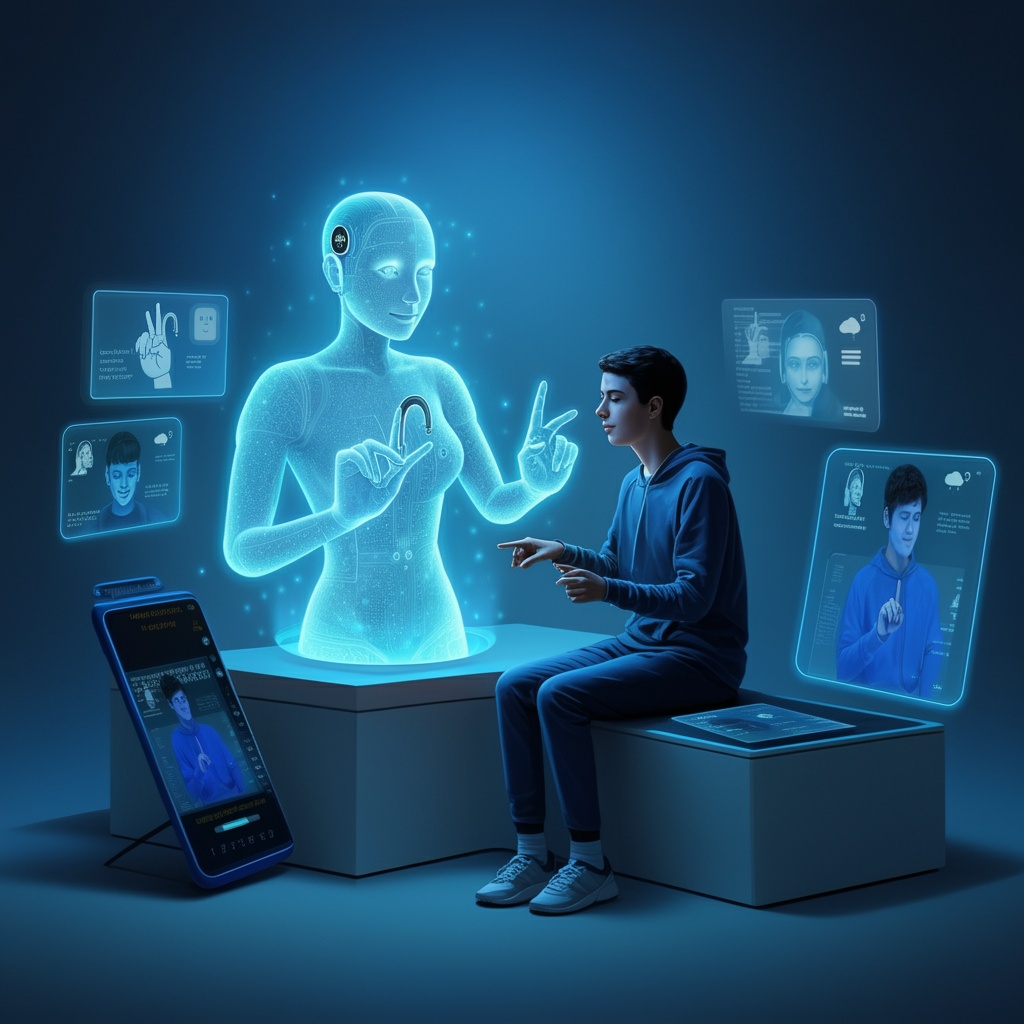
3. AI-Driven Digital Avatars: Translating Sign Language in Real Time
For many in the deaf community, sign language is their primary communication method. However, providing human interpreters for every piece of digital content isn’t always feasible. AI-powered avatars can help fill this gap by offering scalable sign language interpretation.
How Digital Sign Language Avatars Work
- Gathering and Analyzing Data: The first step in creating a digital avatar involves collecting massive amounts of video data that captures not just hand movements but also facial expressions and body language, which are crucial for conveying meaning and tone in sign language.
- Pose Estimation with Computer Vision: AI uses pose estimation techniques to recognize and track critical joints in the signer’s body. Programs like OpenPose or MediaPipe create a skeletal model of the person, which the AI then uses to understand their movements.
- Gesture Recognition with Deep Learning: Once the system has skeletal data, it employs Convolutional Neural Networks (CNNs) to recognize specific gestures by analyzing how joints move over time. These gestures are then labeled with the corresponding sign.
- Applying NLP for Context: Since sign language is heavily context-dependent, the system also uses Natural Language Processing (NLP) to interpret the text or speech input and determine the right signs to use.
- Animating the Avatar in Real Time: Once the system identifies the correct signs, it animates a digital avatar to replicate them. Companies like Robotica are currently working on avatars fluent in British Sign Language (BSL) and expanding to cover other languages, such as American Sign Language (ASL).
Real-World Impact of Digital Avatars
For example, the aiD Project in the European Union developed an on-demand sign language translation app to help deaf users receive signed translations of public announcements or lectures in real time. These avatars can offer consistent and scalable sign language interpretation, making digital content more accessible.
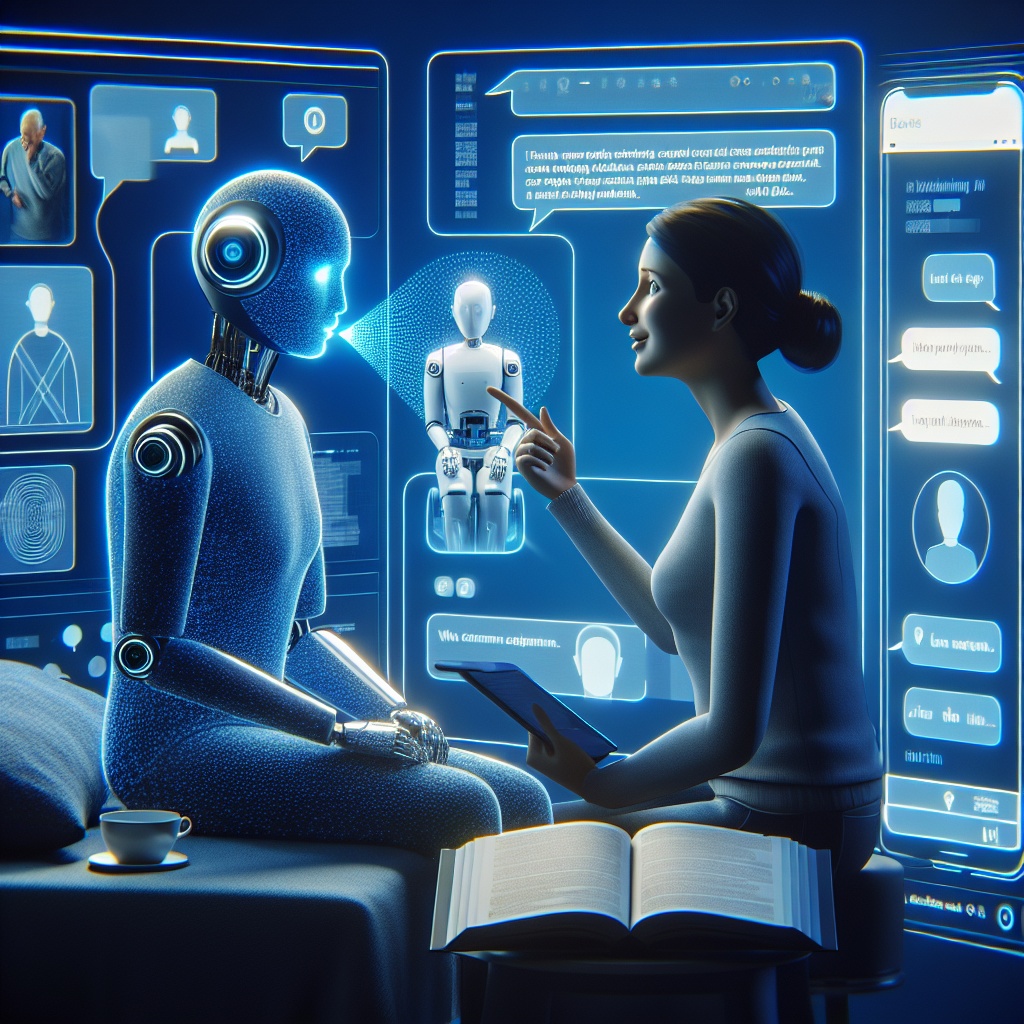
4. Sound Recognition AI: Making Sounds Visible
Sound recognition technology is another area where AI makes a real difference for the deaf community. These systems can alert users to their surroundings in real time by analyzing and identifying essential sounds.
The Technology Behind Sound Recognition
- Training on a Variety of Sounds: The system is trained on large datasets of different types of sounds, including everyday noises like alarms, doorbells, sirens, and even specific events like glass breaking or a baby crying. Each sound in the dataset is labeled with frequency, intensity, and duration metadata.
- Creating Audio Fingerprints: When the system detects a sound, it creates an “audio fingerprint” that captures its unique features. These fingerprints are then compared to a database of known sounds.
- Using Machine Learning for Pattern Matching: AI uses Deep Neural Networks (DNNs) and Convolutional Neural Networks (CNNs) to classify and identify the sound based on its fingerprint.
- Notifying Users in Real Time: When the AI recognizes a sound, it sends an alert to the user’s smartphone or other smart device. The alert could be a visual notification, a vibration, or a signal to a connected smart home system, such as flashing lights.
Real-World Examples of Sound Recognition
Companies like Wavio are developing sound recognition systems that provide visual alerts for essential sounds, such as fire alarms or doorbells. This isn’t just about convenience—it’s about safety and improving quality of life.

Real-World Perspectives: A Visit to the Association of Deaf and Hard of Hearing
While researching the advancements of AI for the deaf and hard of hearing community, I realized that understanding the theory wasn’t enough. I wanted to explore how these innovations were actually impacting people’s lives. So, I reached out to the Association of Deaf and Hard of Hearing in Zagreb, Croatia, where I had the privilege of speaking with two of its remarkable members, Katarina and Iva.
One thing quickly became clear—each person’s experience with deafness or hearing loss is unique, which makes finding a universal solution nearly impossible. What struck me was that the senior members of the Association seemed more enthusiastic about AI advancements than their younger counterparts. Katarina explained that many older members had faced far greater challenges in adapting to society in their youth, and now, they express a deep sense of gratitude for any technology that can make life a little easier. By contrast, younger members, having grown up with more accessible tools, tend to take these advancements somewhat for granted.
Despite these differences, everyone I met uses AI in some form in their daily lives. But there’s a catch—they’ve had to be resourceful. The tools they rely on weren’t originally designed with the deaf or hard-of-hearing community in mind, so they’ve had to creatively adapt existing technologies to meet their needs. The few tools designed specifically for the deaf and hard-of-hearing communities come with hefty price tags, putting a strain on those who need them most.
To make matters worse, the AI-based tools they use often have technical glitches, causing them to crash or malfunction. Each time this happens, valuable time and information are lost, especially when transcribing speech to text. But, as I learned, the deaf and hard-of-hearing community is nothing if not persistent. They continue to experiment and innovate, finding workarounds and solutions where they have yet to officially exist.
Iva shared a story about her college experience that left a lasting impression on me. She described how she used to get through her classes by reading her professors’ lips. However, whenever the professor moved around the classroom or turned away, she was left in the dark, missing key parts of the lecture. Today, the situation is much easier thanks to advancements in speech-to-text technology. Yet, she still feels a pang of sadness when she hears about young people who choose not to pursue higher education, knowing how many obstacles she had to overcome without the tech that now exists.
However, the challenges don’t end at the classroom door. Daily tasks like navigating public transport, scheduling doctor’s appointments, or handling bureaucratic paperwork remain daunting for many. The hearing community rarely even notices these things, but for deaf individuals, they are constant reminders of an inaccessible world.
As I reflected on my conversations, I couldn’t help but think back to the AI technologies I’d explored in theory—innovations with the power to transform lives. But the reality is stark: these advancements aren’t reaching the people who need them most. High costs, technical limitations, and a lack of targeted solutions keep this vital technology out of reach for many. Despite AI’s progress, the tools often feel like they were created with the assumption that everyone experiences deafness in the same way when, in truth, each journey is deeply personal.
It’s ironic and heartbreaking that in a rapidly advancing world with smarter technology, the very people these innovations aim to help are still struggling to be heard. They’re forced to adapt and fight for accessibility in systems that weren’t built with them in mind. It’s not just about creating better tools; it’s about creating a world where everyone has the same opportunity to connect, communicate, and thrive.
As I left the Association, I couldn’t shake the feeling that there’s a crucial message here. AI has come so far, but for those who are deaf or hard of hearing, the silence remains—not from their inability to hear, but from a world that isn’t listening.
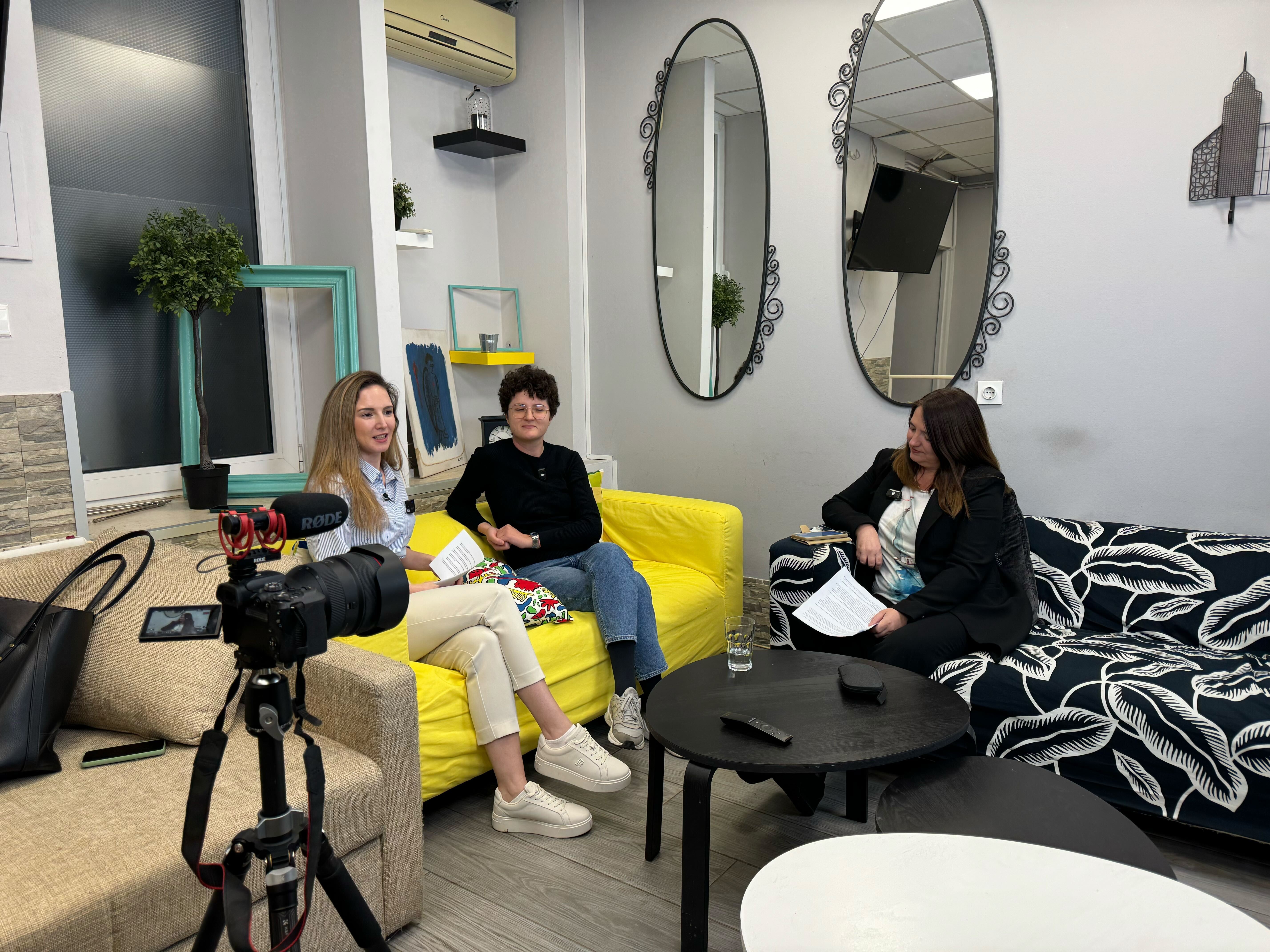