Time-Traveling Through AI: Milestones That Shaped the Future
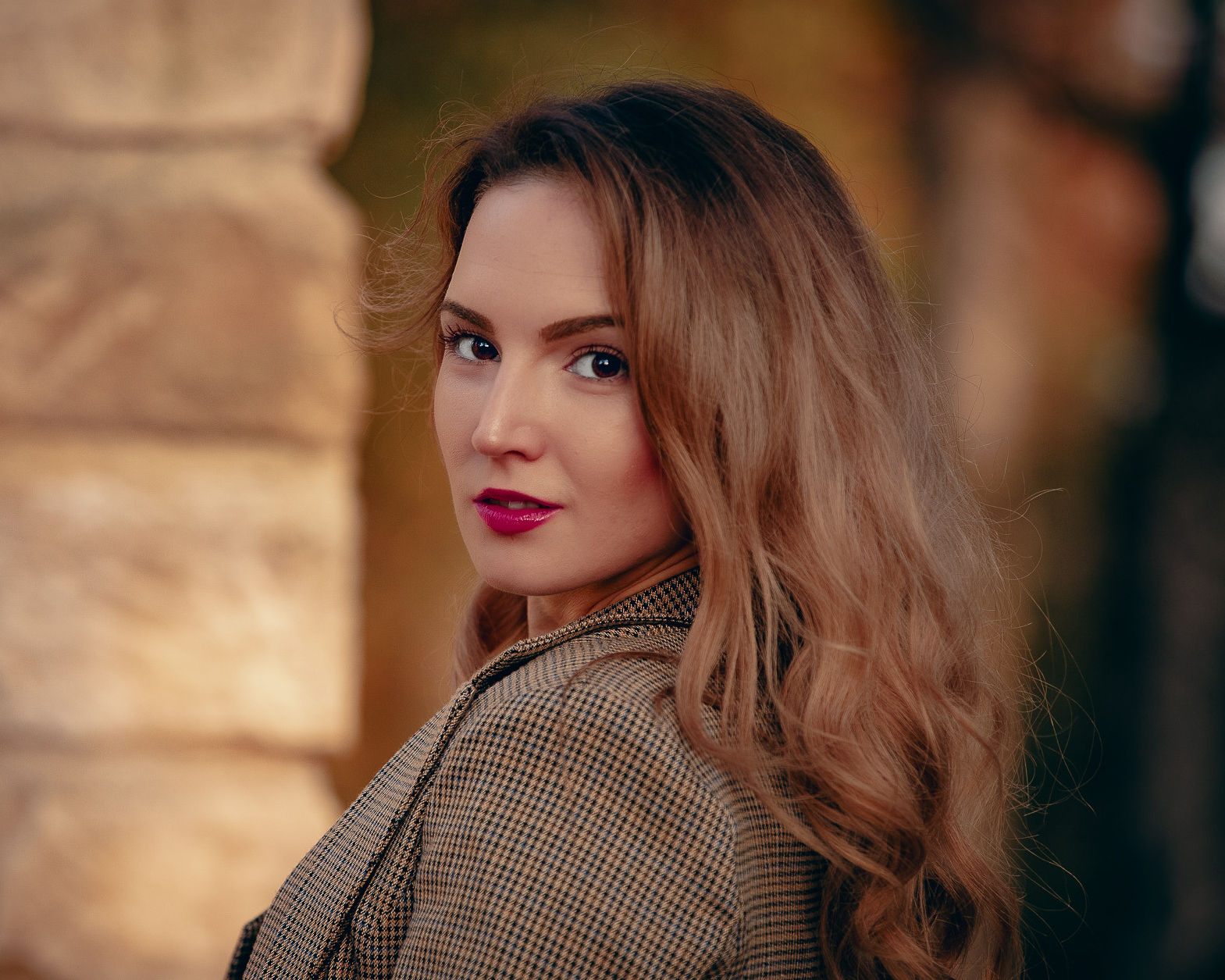
Patricia Butina
Marketing Associate
Published:
January 9, 2025
Topic:
Insights
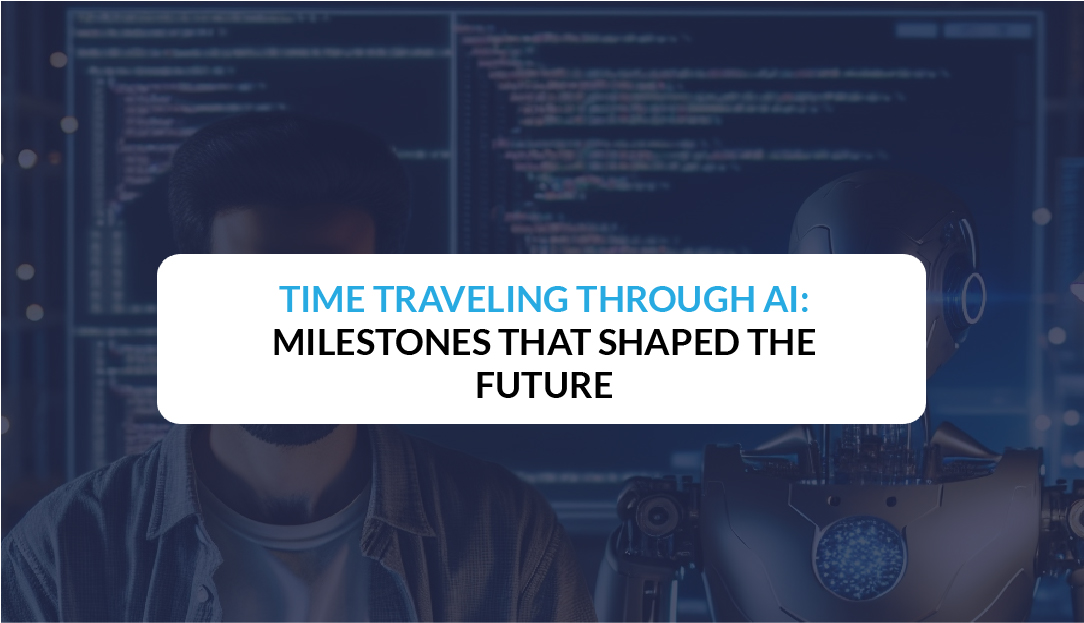
In many ways, artificial intelligence is the story of our desire to build machines that can think, learn, and understand, much like us. It began as an abstract concept in academic discussions and has now evolved into something that powers industries, inspires art, and transforms human lives.
It’s been a remarkable journey. Let’s examine the pivotal moments: brilliance, persistence, and technological breakthroughs that have brought us to where we are today.

1958: The Birth of Neural Networks
Four years after computing pioneer Alan Turing’s death, Frank Rosenblatt introduced the Perceptron, the first neural network model. For its time, it was revolutionary. The Perceptron represented the first step toward machines that could “learn” by mimicking how neurons interact in the brain. It was simple but planted a powerful idea: algorithms that adapt and improve with experience.
This was the earliest glimpse of machine learning. While computational limitations in the mid-20th century constrained its application, the blueprint for neural networks was now available worldwide.

1986: The Backpropagation Revolution
If the Perceptron was the foundation, Geoffrey Hinton, David Rumelhart, and Ronald Williams built the first actual structure with their work on Backpropagation. Backpropagation made it possible to train multi-layer neural networks efficiently, a breakthrough that was decades ahead of its time.
It’s hard to overstate the significance of this. Backpropagation allowed neural networks to learn complex patterns from vast amounts of data, and without it, the modern AI systems we know today would not exist.
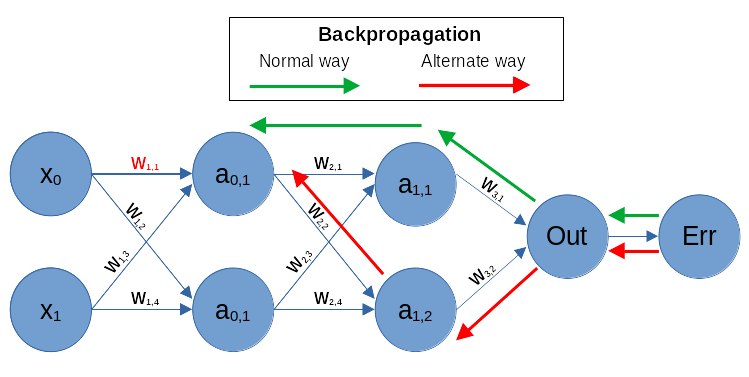
1997: IBM’s Deep Blue Defeats Kasparov
Deep Blue’s defeat of the reigning chess world champion, Garry Kasparov, was a symbolic and technical triumph. A machine could tackle complex, strategic decision.
The victory wasn’t just about chess; it was about proving that machines could outperform humans in areas requiring precision, strategy, and computational power. It made us wonder: What’s next?
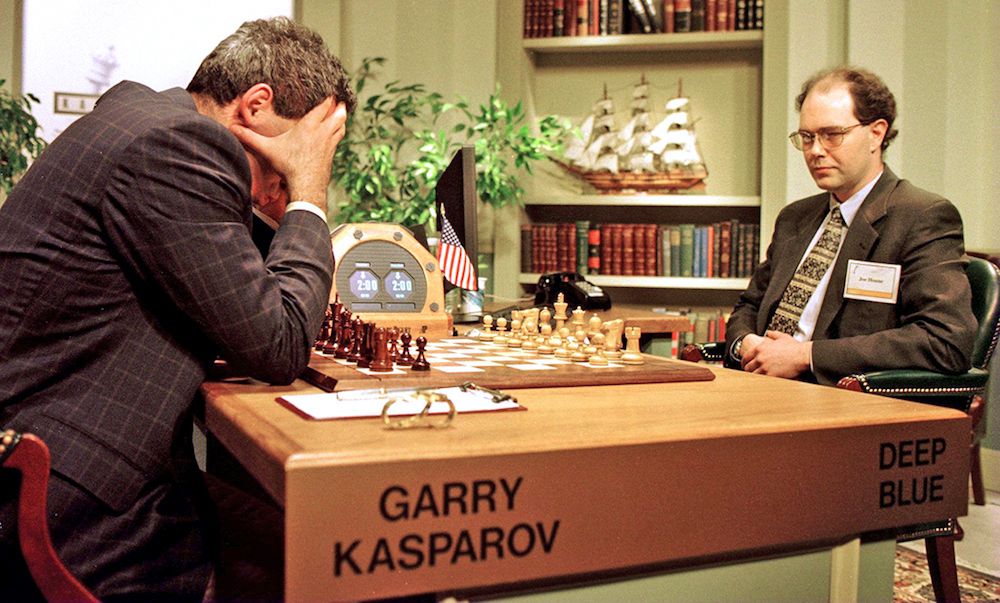
2006: Deep Learning Makes a Comeback
After decades in the shadows, neural networks returned to the spotlight, thanks to Geoffrey Hinton and his team. Their introduction of unsupervised pre-training allowed deeper, more complex networks to train effectively—something that was previously impossible due to computational bottlenecks.
This was the moment when Deep Learning as we know it took shape. Neural networks could now process data across many layers, learning intricate patterns with unprecedented accuracy. It was a pivotal step forward.

2012: The AlexNet Breakthrough
Enter AlexNet—a deep convolutional neural network developed by Alex Krizhevsky, Ilya Sutskever, and Geoffrey Hinton. When AlexNet won the ImageNet competition in 2012, it didn’t just win; it obliterated the competition, achieving results far beyond anything.
This success wasn’t accidental. AlexNet combined deep learning with GPU acceleration, allowing neural networks to train faster and handle much larger datasets. For the first time, AI could genuinely “see” and interpret visual data with a precision that felt almost human. Image recognition was never the same again, and AI’s potential began to feel limitless.

2014: The Advent of Generative AI – GANs
In 2014, Ian Goodfellow introduced Generative Adversarial Networks (GANs), an architecture in which two AIs compete to improve. One generates data while the other critiques it, forcing the first to improve iteratively.
GANs marked the birth of generative AI. Machines could now create—generating realistic images, audio, and even data. It was also the earliest whisper of the generative technologies that power modern systems like ChatGPT and DALL·E.

2017: Transformers and the Power of Attention
Vaswani et al.’s groundbreaking 2017 paper, “Attention Is All You Need,” introduced the Transformer architecture, a turning point.
Transformers brought two key innovations:
● Parallelized computing—enabling faster training on massive datasets.
● Attention mechanisms allow models to “understand” relationships between words, no matter how far apart they appear in a sentence.
Transformers didn’t just change how we build language models; they redefined what was possible in translation, summarization, and understanding context. This brilliant idea is the basis for almost all modern language models, including GPT and BERT.
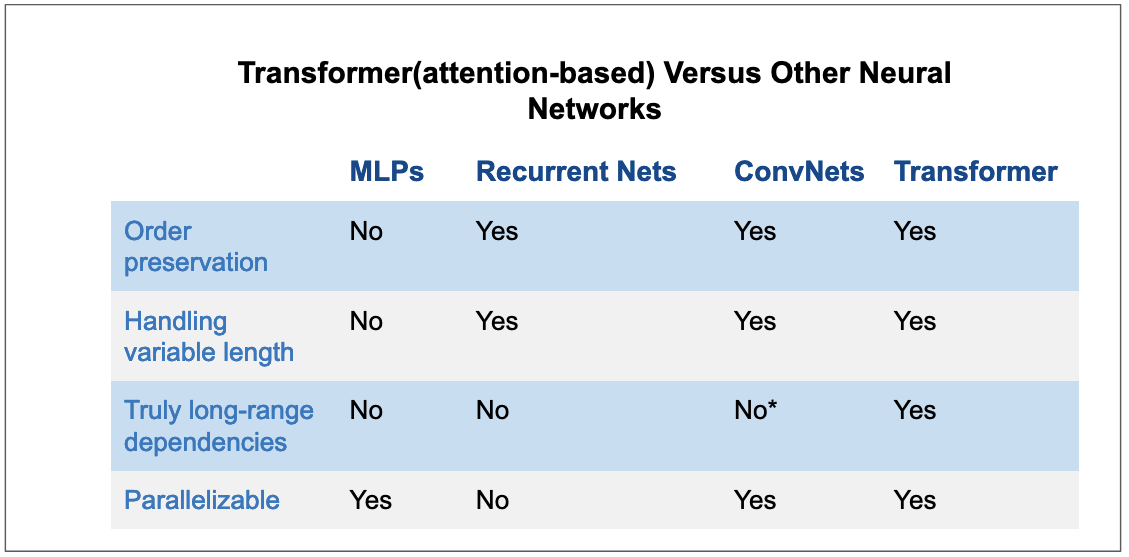
2018–2020: Large Language Models Arrive
By 2018, OpenAI’s GPT (Generative Pre-trained Transformer) set a new benchmark for language models. With vast amounts of text data and a Transformer-based architecture, GPT demonstrated how AI could understand, generate, and respond to natural human language. Then, in 2020, GPT -3 came along. With 175 billion parameters, it pushed the boundaries of coherent, intelligent language generation. For the first time, conversational AI felt “human.” It could write essays, answer questions, and even create poetry.

These models were technological marvels and foundational tools that unlocked new possibilities for businesses, creators, and developers worldwide.
2022: Multimodal AI – DALL·E and Beyond
In 2022, OpenAI’s DALL·E extended generative models beyond language. It allowed users to create images from simple text prompts, showcasing AI’s ability to work across text, photos, and more modalities.
This wasn’t just about pretty pictures; it demonstrated AI’s deep understanding of concepts, relationships, and creativity.
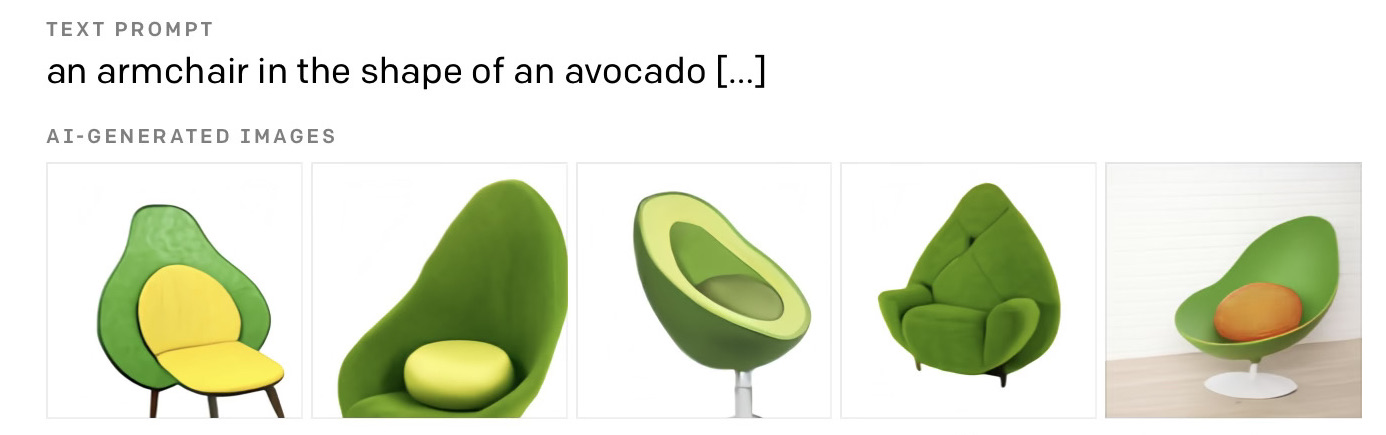
2022–2023: ChatGPT and the Generative AI Explosion
Then, on November 30, 2022, ChatGPT 3.5 entered the world—and AI moved from labs to the mainstream. For the first time, millions of people could interact with advanced generative models that were accessible, intuitive, and—most importantly—useful.
From businesses streamlining workflows to individuals using AI for creativity and learning, the explosion of generative AI is reshaping how we work, think, and live.

What’s Next?
From the Perceptron in 1958 to today’s generative models, the story of AI has been one of relentless progress, driven by brilliant minds, computational power, and an ever-expanding understanding of how machines can learn.
But we’re just getting started. The next breakthroughs will push the boundaries even further, creating systems that are smarter, more capable, and more aligned with human needs.
One thing remains clear: AI is a tool for possibility, a partner for innovation, and a force for positive change.