More Than Meets the AI: The Multimodal AI Revolution
No items found.
Published:
October 28, 2024
Topic:
Insights

Artificial Intelligence has taken enormous strides in recent years, but there's a shift happening that will change the way machines understand the world: multimodal AI. While traditional AI systems rely on a single type of data—whether it's text, images, or sound—multimodal AI brings together multiple data types, processing them simultaneously for richer, more accurate insights.
We’re moving toward a future where machines not only see or hear but can truly understand complex, multi-layered contexts, similar to how humans do. Imagine an AI that can watch a video, listen to the dialogue, and read a subtitle simultaneously—and make sense of it all.
Let’s dive deep into how this works, and why it’s such a big deal.
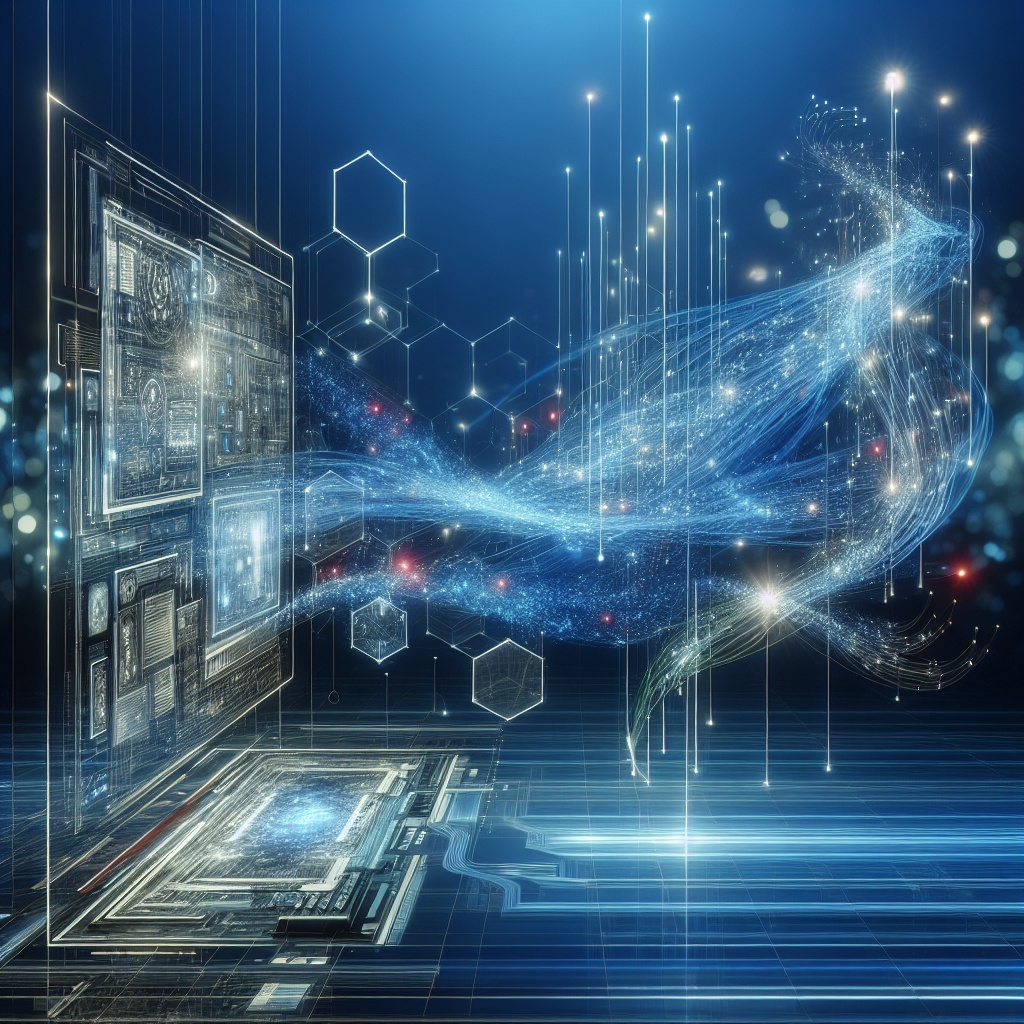
What is Multimodal AI?
At its core, multimodal AI refers to artificial intelligence systems capable of analyzing and integrating multiple types of data (known as modalities) simultaneously. Whether it’s text, images, audio, or sensory input, multimodal AI processes all of these at once, delivering outputs that reflect a more holistic understanding.
This concept is inspired by how humans naturally interact with the world. For example, when you’re watching a video, you don’t just process what you see. You’re listening to the sound, understanding the speech, and interpreting the overall context—all at the same time. Multimodal AI seeks to replicate that layered cognitive process.
Why Does It Matter?
Single-modal AI has its limitations. If an AI can only process text or images in isolation, it lacks the ability to understand the broader context that would be obvious to a human. Multimodal AI, on the other hand, allows machines to understand the full picture by pulling together multiple streams of information. This leads to better decision-making and enhanced performance in areas like self-driving cars, healthcare diagnostics, and natural language understanding.

How Multimodal AI Works: A Closer Look
Let’s get technical. Understanding how multimodal AI functions requires breaking down the processes involved in integrating various forms of data. Here are the key components:
1. Modality-Specific Processing
The first step in a multimodal AI system is having modality-specific models. These are specialized networks designed to handle a specific type of input:
- Convolutional Neural Networks (CNNs) for images and video,
- Transformer models (like BERT or GPT) for text,
- Recurrent Neural Networks (RNNs) for sequential data, such as audio.
Each modality-specific network extracts meaningful features from its input data. For instance, a CNN would analyze an image and identify important features like shapes, objects, and colors. A transformer model analyzing text would focus on extracting semantic relationships, such as word meanings and sentence structures. Audio models extract features like pitch and rhythm to understand speech or sound patterns.
The result of this stage is a set of feature vectors, which are numeric representations of the key elements in each input. These feature vectors will eventually be combined or “fused” to allow the AI to understand the whole context.
2. Cross-Modal Attention and Alignment
Next, we have the process of cross-modal alignment. This is where the magic really starts to happen. Since each type of data (text, images, audio) has its own structure and format, we need a mechanism to align these modalities so that they can be meaningfully integrated.
This is typically done through attention mechanisms, popularized by transformer models like BERT and GPT-3. Attention mechanisms allow the model to focus on the most relevant parts of the data across different modalities.
Imagine watching a movie and reading subtitles at the same time. You’re naturally focusing on both what’s happening on-screen and the text to interpret the full context. Multimodal AI uses attention mechanisms to perform a similar function—matching relevant parts of different data types and linking them together. For example, if an AI is watching a video, attention will help it correlate an object in the frame with the word that describes it in the accompanying audio.
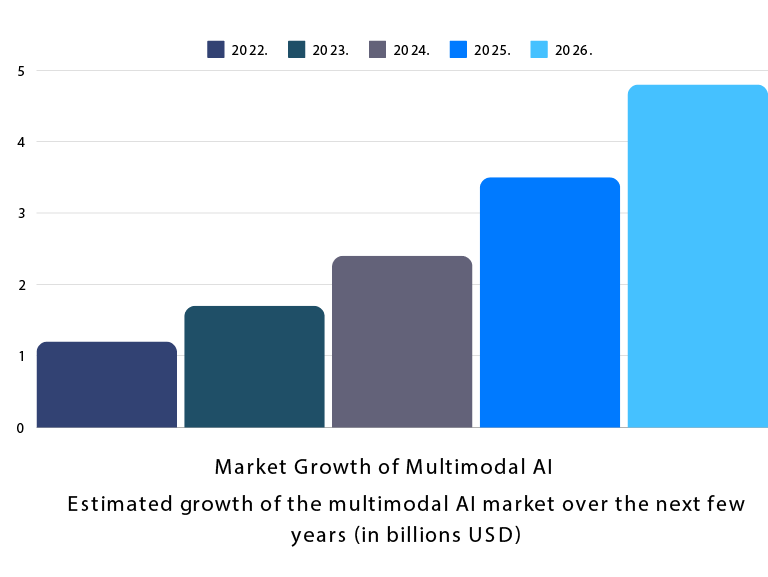
3. Feature Fusion
Once the features from each modality are extracted and aligned, they are combined through feature fusion. The fusion process integrates the various feature vectors from different modalities into a single, unified representation. There are several approaches to fusion, each suited to different types of tasks:
- Early fusion: The features from all modalities are merged at an early stage, allowing the system to learn from the combined data.
- Late fusion: Each modality is processed separately, and their outputs are combined later, often used when the data streams are relatively independent.
- Hybrid fusion: A combination of both early and late fusion, allowing certain features to be integrated early while others are fused later.
The goal here is to create a comprehensive understanding of the input data, allowing the system to make decisions that take all relevant information into account.
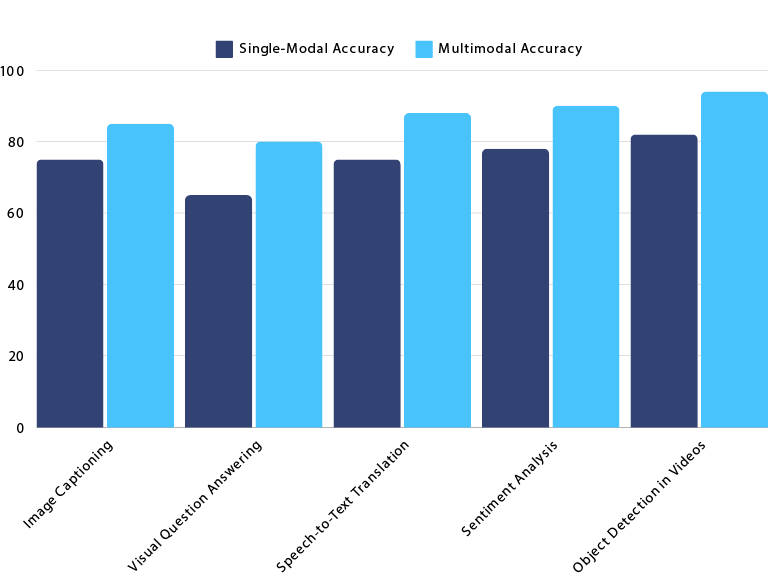
4. Decision-Making and Output
Finally, after fusing the multimodal data, the system moves on to the decision-making phase. Depending on the task, the AI system will either provide predictions, generate output (like a caption for an image or a transcript for a video), or take action (in the case of self-driving cars or robotics).
For example:
- In a medical diagnosis system, multimodal AI can analyze an MRI scan and cross-reference it with patient history and lab reports to provide a more accurate diagnosis.
- In autonomous vehicles, the AI integrates visual data from cameras with LIDAR and radar to decide whether to stop, accelerate, or turn.
Real-World Applications of Multimodal AI
Now that we’ve covered how it works, let’s talk about where multimodal AI is already having an impact.
1. Healthcare and Diagnostics
In healthcare, multimodal AI systems are transforming how diagnoses are made. By integrating medical images (like X-rays or MRI scans) with textual data (patient history, doctor’s notes), these systems can provide much more accurate and comprehensive insights. This approach reduces human error and leads to faster, more reliable diagnostic results.
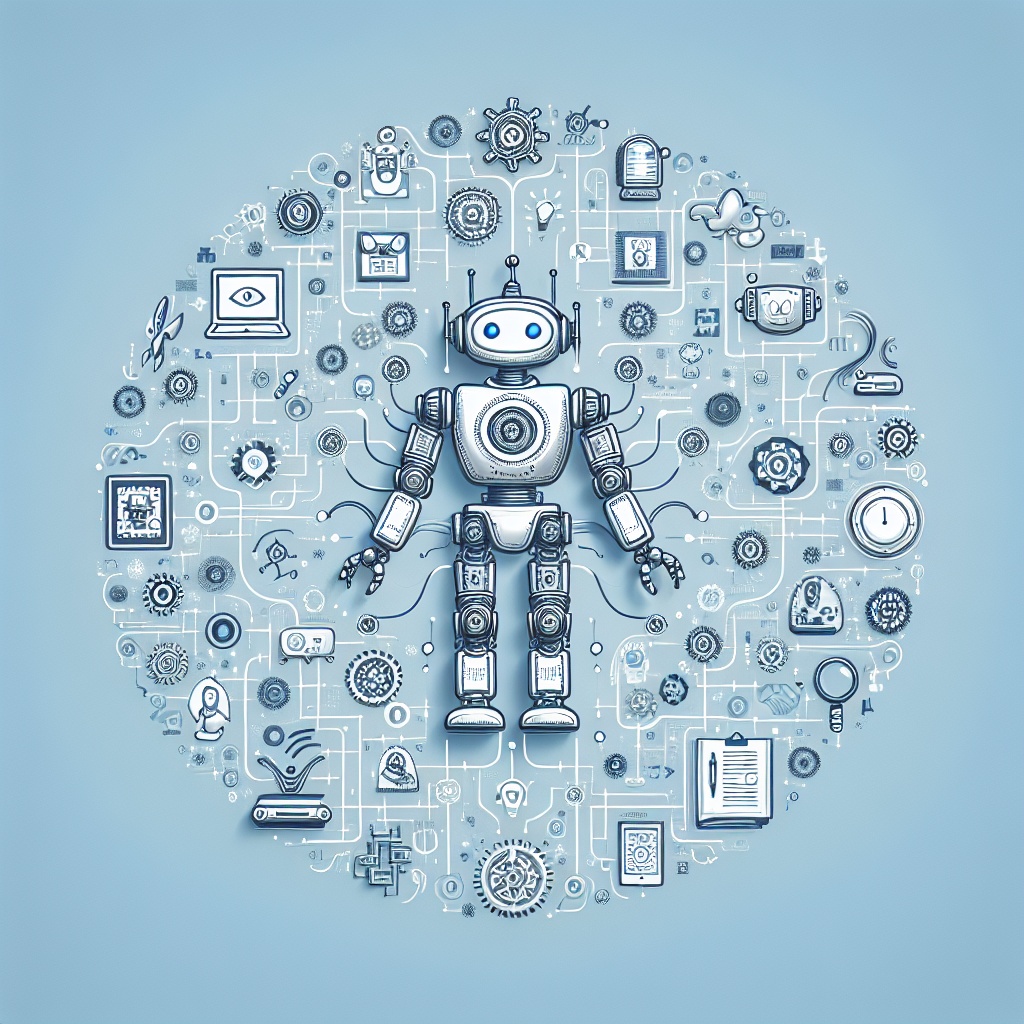
2. Autonomous Driving
Self-driving cars depend heavily on multimodal AI to interpret their surroundings. These vehicles combine inputs from cameras (to detect visual information like road signs), LIDAR (to map out distances and obstacles), and radar (to understand speed and movement). The fused data gives the car a full view of its environment, allowing it to navigate safely.
3. Natural Language Processing (NLP)
In conversational AI, multimodal systems are being used to improve how virtual assistants or chatbots interact with users. Imagine a virtual assistant that can not only understand your spoken commands but also read visual clues or text inputs—creating a more intuitive and responsive interaction.
4. Media and Content Creation
Multimodal AI is also breaking new ground in the world of media. Systems that analyze video content are now capable of generating subtitles, descriptions, or even editing suggestions by combining audio, visual, and textual inputs. This is a game-changer for content creators, making it easier to produce accessible media and even automate tasks that previously required manual intervention.
.png)
Challenges in Multimodal AI
For all its potential, multimodal AI still faces several challenges. Aligning different types of data (like matching text with images or sound) is no easy task, particularly when dealing with large, unstructured datasets. Additionally, training these models requires massive computational power and huge volumes of labeled data, which can be expensive and time-consuming to gather.
Another major hurdle is the interpretability of multimodal models. As these systems become more complex, understanding how decisions are made (and ensuring they are free from bias) becomes a critical issue, particularly in fields like healthcare or law, where decisions have life-changing consequences.
The Future of Multimodal AI: What’s Next?
The future of AI is undeniably multimodal, and we’re only scratching the surface of its potential. In the coming years, we can expect to see multimodal systems become even more integrated into everyday applications, enhancing everything from education to entertainment, and even how we interact with technology in our homes.
One of the most exciting areas of research is self-supervised learning, where AI systems can learn to understand and align multimodal data without the need for extensive labeled datasets. This would dramatically lower the barrier to training these models and speed up the development of even more advanced systems.
Moreover, we’ll likely see advancements in human-computer interaction, where multimodal systems can understand not only what we say but how we say it, combining our words, gestures, and even facial expressions to create a more natural and fluid interaction.
Conclusion
Multimodal AI is changing the landscape of artificial intelligence, making machines more capable of processing and understanding data the way humans do. By fusing text, images, audio, and more, multimodal systems provide a richer, more contextual understanding of the world. Whether it’s in autonomous vehicles, healthcare, or natural language processing, this technology is setting the stage for smarter, more versatile AI systems.
As the technology matures, the possibilities for multimodal AI are practically limitless, and it’s only a matter of time before it becomes a standard in various industries. Keep an eye on this space—there’s much more to come.